Several times a day I find myself frozen in front of a dropdown menu—should I use Sonnet or Haiku? GPT-4o or GPT-4o with scheduled tasks? Or perhaps o1? o3-mini? o3-mini-high? The list goes on. Despite spending hours with these tools daily, I can’t shake the feeling that I’ve wandered into a developer meeting I wasn’t invited to.
I’m not alone. Earlier this month, Julian Lehr, a writer at Linear, tweeted: “There’s a huge market opportunity for a brand agency that helps AI companies with model naming.” Product designer Sam Henri Gold posted: “Old joke about how naming things is hard but my god is there a gas leak in these offices.”
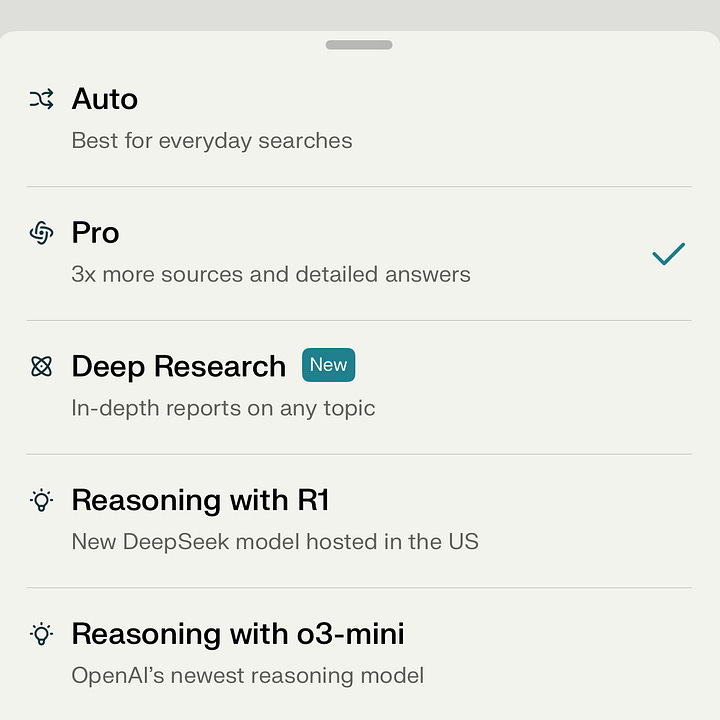
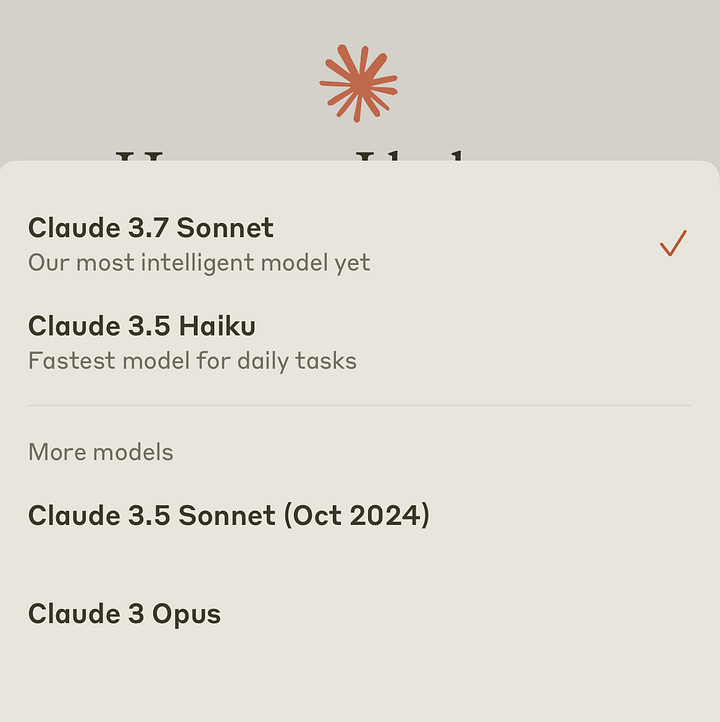
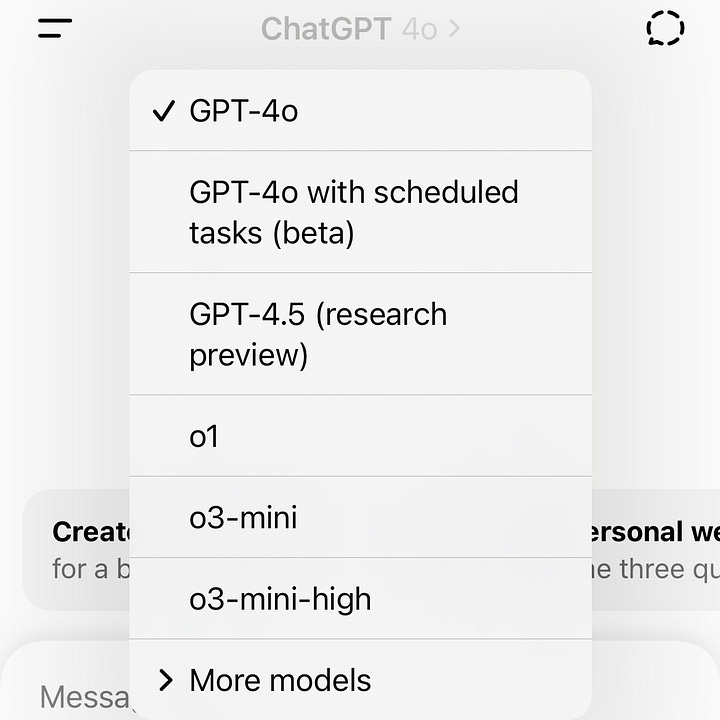
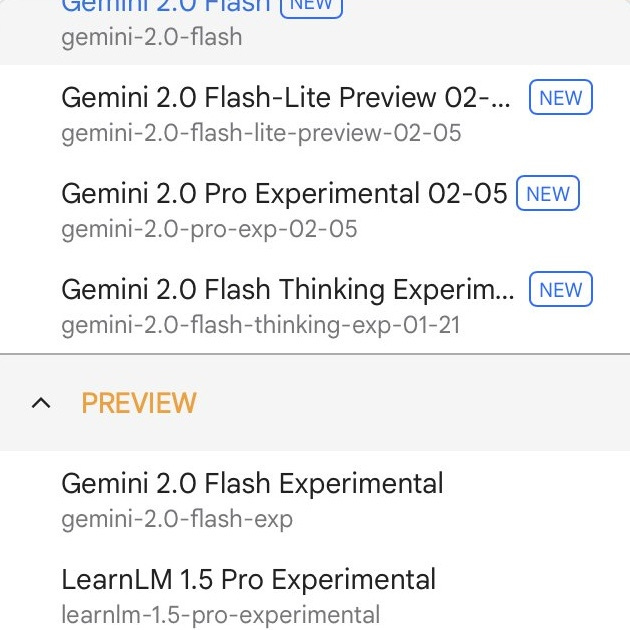
But this isn’t just about branding. AI naming is a UX problem. The names we give these systems shape how people understand them—what they do, how to choose between them, and who they’re for. When naming is unclear or overly technical, it reinforces the idea that these tools aren’t for most people. In an era where AI promises to transform how we work, inscrutable naming conventions create friction that keeps these tools out of the hands of those who may benefit most.
Why is AI naming so confusing?
If these naming schemes create such barriers, why do companies persist with them? Several factors might explain this seemingly deliberate complexity:
Signaling technical prowess. Acronyms and code-like naming (e.g. o3-mini-high) project complexity. The cryptic command-line aesthetic harkens back to the early days of computing when tech was the domain of specialists—and keeps it that way.
Creating an aesthetic of progress. Version numbers and incremental designations (3.5, 3.7, etc.) create the impression of continuous improvement—important in the AI space race where companies compete for funding and market dominance.
Maintaining flexibility. Vague naming schemes allow companies to pivot product positioning without changing established names.
Avoiding direct comparisons. If everyone used intuitive, function-based naming, direct comparisons would be easier. By keeping names obtuse, companies make it harder for consumers to make apples-to-apples comparisons.
AI naming mirrors the current state of AI itself: fast-moving, experimental, insider-driven, and not quite ready for mass adoption. But if AI is going to live up to its promise, naming can’t just serve its makers; It has to serve the people using it.
The historical context of terrible tech naming
Tech has always had a naming problem. As Roon once put it: “You can either work for a liberal tech company that lifted its name off of a random concept in Hinduism, or you can work for a conservative tech company that lifted its name off a random concept in Tolkien. Those are your choices.”
This tracks. Elaine Moore at Financial Times notes that “incoherent nomenclature is a tradition in the tech sector, where titles are often designed to amuse teams, not users.”
AI has continued the tradition: opaque, functional, and forgettable on one side (GPT-4o, DeepSeek R1, Llama 3.3), and grandiose myth-making (Gemini, Claude, Grok) on the other. AI naming has unraveled into a landscape of inconsistent logic, vague modifiers, and too many choices. Even insiders need cheat sheets to keep up.
AI models vs. AI interfaces
Another source of confusion? Most companies don’t clearly distinguish between AI models (the tech) and AI interfaces (how people use the tech). When you use ChatGPT, you’re not talking to GPT-4 directly—you’re using an interface built on top of it. But at Google, Gemini can refer to both assistant and model.
It gets messier: startups are layering their own apps on top of these LLMs, too, creating a new “application layer” or “wrapper” with routing, reasoning, and retrieval capabilities (RAG). As Sequoia’s Sonya Huang and Pat Grady wrote: “Application layer AI companies are not just UIs on top of a foundation model.” They’re full-stack experiences. But we’re still labeling them like firmware updates.
The chat interface itself isn’t intuitive for most people. Prompting is a skill—one that power users have developed over time. For everyone else, it’s a barrier to entry.
How to fix it
According to Onym—legendary naming resource from
and Greg Leppert—great names do two things: communicate value and stick in your memory. AI companies could take a few cues from that:Separate internal versioning from public naming. No one markets an iPhone by its processor model. Let the backend stay in the backend.
Create a clear hierarchy. Anthropic’s Haiku/Sonnet/Opus trio is a good start. Think: good / better / best or basic / standard / pro.
Stick to one naming system. Whether you use numbers, names, or metaphors—consistency matters.
Focus on function, not specs. Most users don’t care about parameter counts or training methods—they care about what the AI can do for them. Name and present options based on capabilities, not technical details.
Test with real users. This seems obvious, but I’m convinced some of these names never made it past the engineering Slack. Show your naming system to non-technical users and see if they can intuit what each option means.
And my favorite: In a crowded market, memorability matters more than descriptiveness. Aim for distinctiveness.
The last word
Naming might seem superficial compared to the technical complexity behind AI, but it’s the first interaction people have with the product. Get the name right, and you win. Get it wrong, and you leave users hovering, uncertain, frustrated.
Until someone fixes this, I’ll be here, hovering over o3-mini-high, wondering if it’s better than o3-mini—and whether either is what I actually need.
Someone please open a window in these naming meetings. The industry deserves better. And so do we.
—Carly
If you’re new here (or just confused), here’s how a few major players are approaching model and assistant naming:
OpenAI, creators of ChatGPT, started simple with GPT-1 through GPT-4. Easy enough. Now? We’re choosing between GPT-4o (multimodal), o1 (smart), o3 (smarter), o3-mini (smaller), and o3-mini-high (??).
Google’s assistant began as Bard and was later rebranded to Gemini, which operates on models like Gemini Pro, Ultra, Nano, 1.5, and 1.5 Flash. They also have the Gemma family, with variants like CodeGemma and PaliGemma.
Meta took the acronym route with LLaMA (“Large Language Model Meta AI”), currently at LLaMA 3.1, 3.2, 3.3 accessible through Meta AI.
Microsoft skipped the chaos by calling everything Copilot. It’s a clear metaphor—assistant, not overlord—but applied so broadly (GitHub, Office, Windows) that it’s hard to tell which Copilot does what.
Apple, ever the branding experts, rolled out Apple Intelligence—a clean play on “AI” that slots perfectly into their product naming system. “Putting it all under a single obvious, easily remembered—and easily promoted—name makes it easier for users to understand,” wrote John Gruber in the only positive line of an otherwise scathing review of the tech.
DeepSeek didn’t bother with metaphor. Just V2, V3, R1. You know where you are in the version history, even if you have no idea what the model actually does.
Anthropic personifies their assistant Claude, and uses a poetic model hierarchy: Haiku (lightweight), Sonnet (medium), Opus (powerful). The naming is thoughtful, even charming—until you get into versions like Claude 3.7 Sonnet and 3.5 Haiku.
“Bard with x model” actually made a lot of sense to me! (still miss ya, Bard)!
most of the time i care very little about what something is called. what bugs me is when a product doesn’t explain itself, what it can do and can I use it for free (at least til I can see the value in paying). that’s still the opportunity for UX and content design, to guide users to what they need and are willing to pay for. I’m not hopeful we can turn the tide of model naming nerdery but we can at least remember users are people, not computers ¯\_(ツ)_/¯
I wish the models UI reflected what they are useful for. Like you said "The chat interface itself isn’t intuitive for most people. Prompting is a skill—one that power users have developed over time. For everyone else, it’s a barrier to entry."
The chat interface feels very terminal inspired!
What if we have specific models named for specific tasks e.g. "Journal" "Day planner" "Image" that then took people through flows which asked them questions to help get to a more desired outcome. It's too open ended right now.